Addressing Membership Inference Attack in Federated Learning with Model Compression
Authors: Németh, G. D. , Lozano, M. A. , Quadrianto, N. , Oliver, N.
External link: https://arxiv.org/abs/2311.17750
Publication: arXiv preprint:2311.17750, 2023
DOI: https://arxiv.org/abs/2311.17750
Federated Learning (FL) has been proposed as a privacy-preserving solution for machine learning. However, recent works have shown that Federated Learning can leak private client data through membership attacks. In this paper, we show that the effectiveness of these attacks on the clients negatively correlates with the size of the client datasets and model complexity. Based on this finding, we propose model-agnostic Federated Learning as a privacy-enhancing solution because it enables the use of models of varying complexity in the clients. To this end, we present MaPP-FL, a novel privacy-aware FL approach that leverages model compression on the clients while keeping a full model on the server. We compare the performance of MaPP-FL against state-of-the-art model-agnostic FL methods on the CIFAR-10, CIFAR-100, and FEMNIST vision datasets. Our experiments show the effectiveness of MaPP-FL in preserving the clients’ and the server’s privacy while achieving competitive classification accuracies.
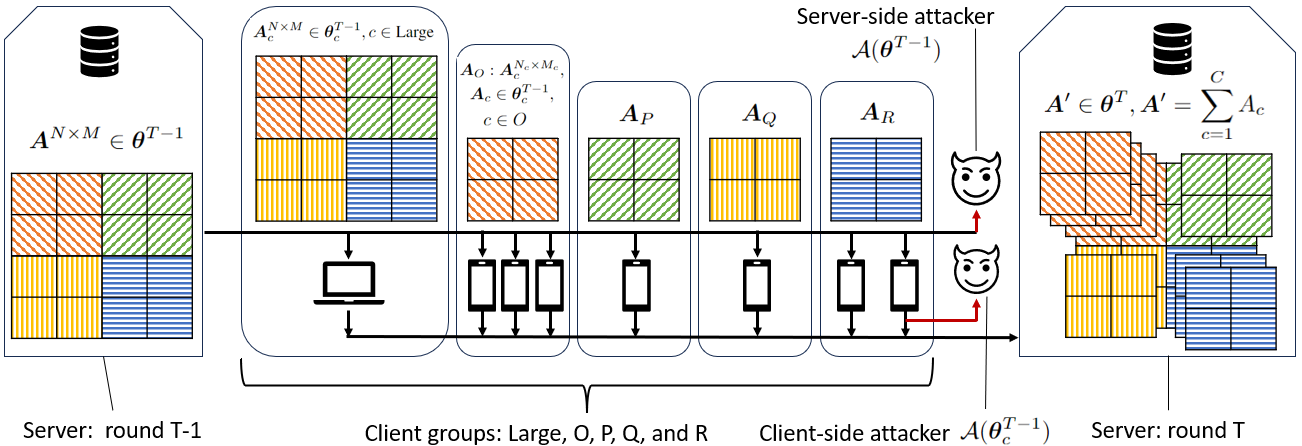