The Beautyverse
AI-enhanced face filters are becoming increasingly pervasive on social media platforms. These filters leverage algorithmic advances in Computer Vision to automatically detect the face and facial features of the user, and Computer Graphics (namely Augmented Reality or AR) to superimpose digital content in real-time, enhancing or distorting the original facial image.
Different types of AR face filters are currently available on social media for a variety of applications and use cases. In recent years, AR face filters are increasingly used to beautify the original faces and make them conform to certain canons of beauty by digitally modifying facial features. We refer to these filters as beauty filters; and we refer to the set of self-representation aesthetic norms that they carry as the BeautyVerse.
Nowadays, thousands of AR beauty filters are available on Instagram and other social media platforms. These filters apply similar transformations to the input image: they provide a smooth and uniformly colored skin, almond shaped eyes and brows, full lips, a small nose and a prominent cheek structure.
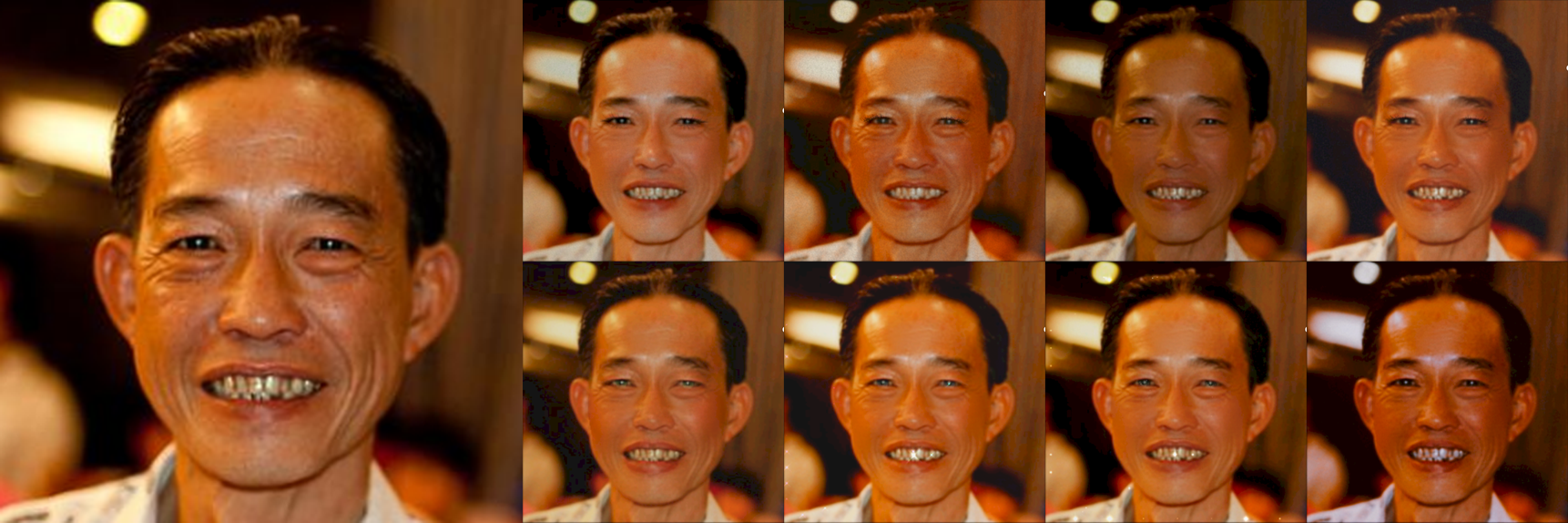
Thanks to our framework “OpenFilter”, we have created two publicly available datasets (namely FairBeauty and B-LFW) to computationally investigate the characteristics of the Beautyverse. FairBeauty is the beautified version of FairFace, i.e. a dataset that is diverse by design, considering age, gender, race, position and other features. B-LFW is, instead, a beautified version of LFW, a benchmark dataset for face recognition. More information about the framework and the datasets are available on the OpenFilter Datasets page.
Our current experimental outcomes include:
- the homogenization of aesthetics of beaty filters;
- the impact of beauty filters on face recognition techniques;
- the presence of racial biases in popular beauty filters.
1. Homogenization of aesthetics
We hypothesize that beauty filters homogenize facial aesthetics making the beautified faces more similar to each other. As previously stated, the images in FairFace are diverse by design. In this experiment, we aim to assess whether the application of beauty filters reduces the diversity. We consider both the FairFace and the FairBeauty datasets. We conduct this experiment using the six different models, i.e. DeepFace, VGG-Face, Facenet, CurricularFace, MagFace and ElasticFace. We compute the distances between a different subset of 500 pairs of images, so that the overall measurements consider 3,000 distinct pairs of images, to minimize potential biases in the results. We evaluate the homogenization using the average distance of all sampled pairs from FairFace and FairBeauty datasets, i.e. the lower the average distance, the greater the homogenization.
As a reference, we perform the same computation when applying Gaussian filtering (blurring) and down-sampling (pixelation) to the original faces of the FairFace dataset. This comparison allows a better understanding of the potential diversity loss due to the beauty filters.
The results of this experiment are shown in the Figure below.
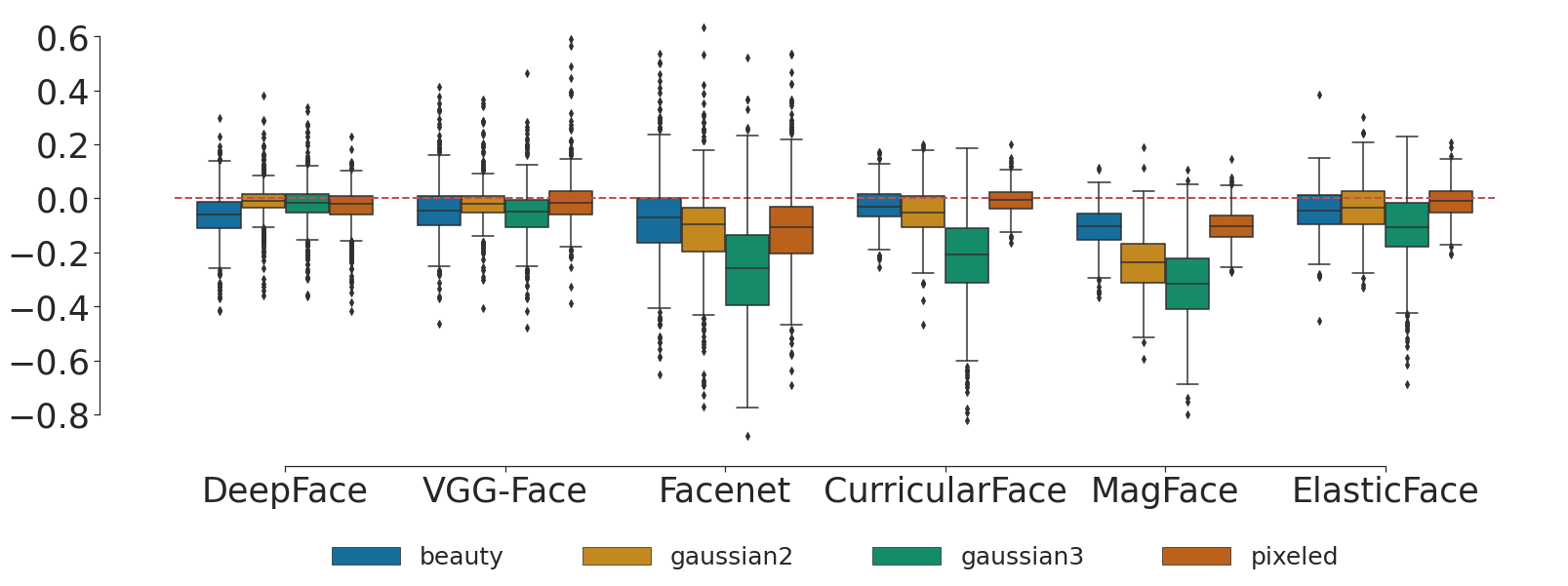
For each pair, distances between transformed images are plotted in terms of differences w.r.t. the distance between the original images. A value of 0 (plotted as a dashed red line in the Figure) means that there is no difference between the original distance and the distance after applying one transformation, i.e. the transformation does not affect the distance between the faces. In all cases, the measurements obtained on the beautified version have lower average distance than those of the original dataset. In other words, according to these experiments, the beautified faces in FairBeauty are statistically more similar to each other than the original faces.
2. Impact on Face Recognition
We evaluate the performance of three state-of-the-art face recognition models (CurricularFace, ElasticFace and MagFace) on the original LFW dataset, on each single beauty filter applied to LFW and on the B-LFW dataset (in which different beauty filters are applied on different images of the same individual). To perform these experiments, we filter the entire LFW dataset with each of the filters, creating eight different variants of it, one for each beauty filter. The obtained results are shown in the Table below.
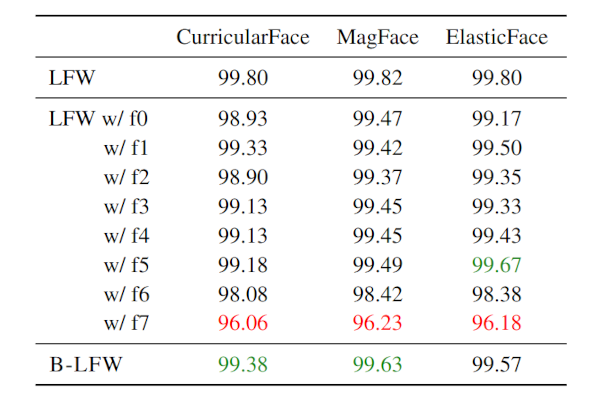
We observe that the results on B-LFW do not show a significant decrease in the performance of state-of-the-art face recognition models.
3. Racial bias
In our experiments, we investigate whether beauty filters implicitly make beautified individuals of all races look whiter. To tackle this question, we leverage two state-of-the-art race classification algorithms. The faces in FairFace are labeled according to seven different racial groups, namely: Black, East Asian, Indian, Latino Hispanic, Middle Eastern, Southeast Asian, and White. In our experiments, we randomly sample a subset of 5,000 faces for each race. We compare the performance of the race prediction algorithms on the face images from FairFace and the corresponding beautified version in FairBeauty.
The predicted value of the label White significantly increases in the beautified faces of all races when compared to the original, non-beautified images. Moreover, there is a significant loss in the performance of the race classification algorithm when applied to the beautified faces of most races except for the images labeled as Whites, whose performance increases in the beautified version of the original faces. In other words, there is a larger probability to classify the beautified faces –independently of their race– as white.
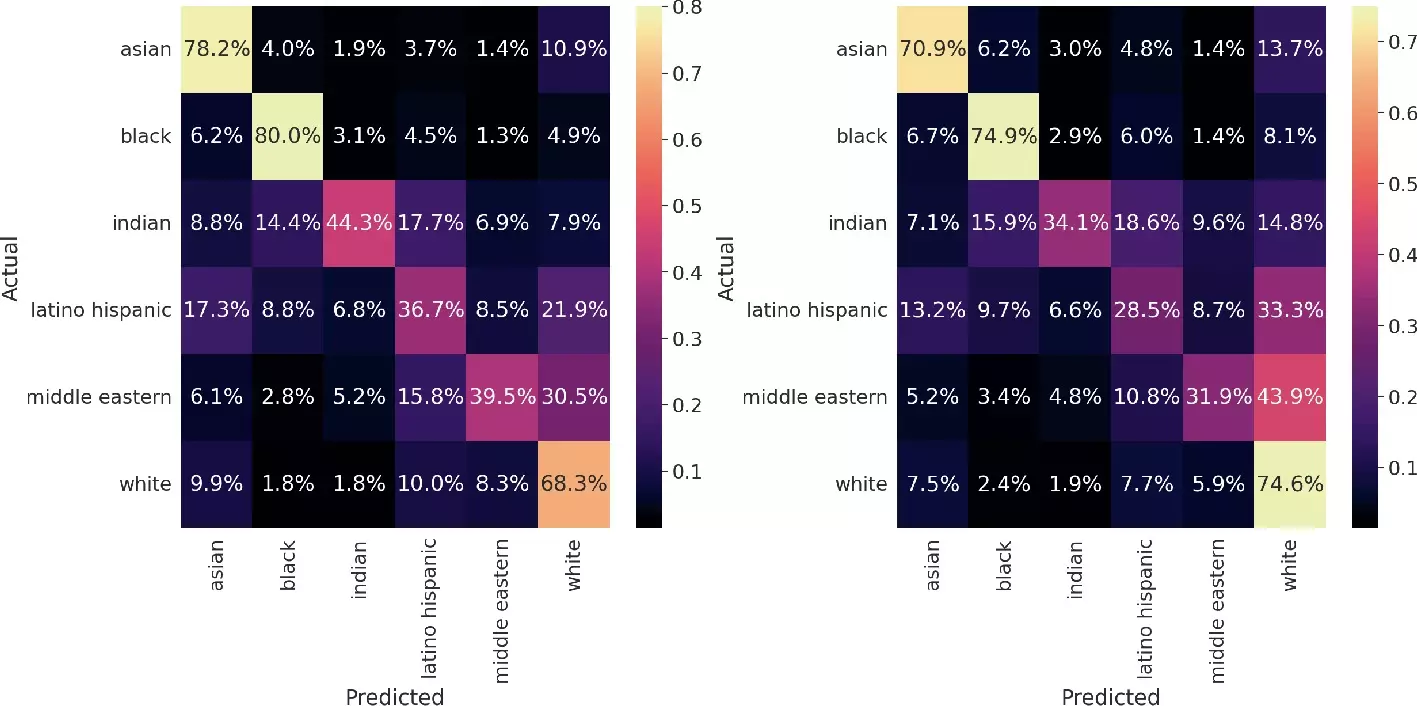
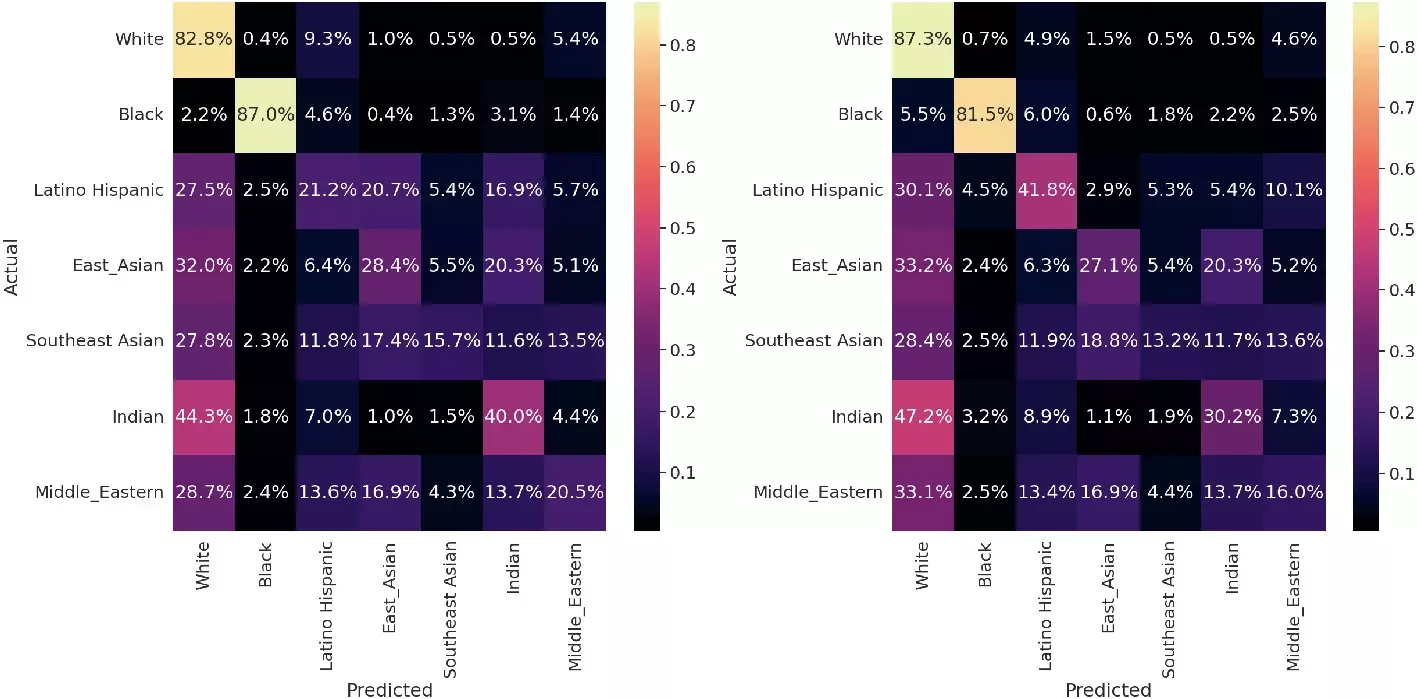
Our work in the media
Levante: El sesgo racista de los filtros de belleza de Instagram y Tik Tok
Diario de Mallorca: El sesgo racista de los filtros de belleza de Instagram y Tik Tok
ELLE: Nuria Oliver: “Los algoritmos definen la belleza”