OpenFilter: A Framework to Democratize Research Access to Social Media AR Filters
Authors: Riccio, P. , Psomas, B., Galati, F., Escolano, F. , Hofmann, T. , Oliver, N.
External link: https://arxiv.org/abs/2207.12319
Publication: 36th Conference on Neural Information Processing Systems (NeurIPS) Datasets and Benchmarks Track, 2022
DOI: https://doi.org/10.48550/arXiv.2207.12319
Augmented Reality or AR filters on selfies have become very popular on social media platforms for a variety of applications, including marketing, entertainment and aesthetics. Given the wide adoption of AR face filters and the importance of faces in our social structures and relations, there is increased interest by the scientific community to analyze the impact of such filters from a psychological, artistic and sociological perspective. However, there are few quantitative analyses in this area mainly due to a lack of publicly available datasets of facial images with applied AR filters. The proprietary, close nature of most social media platforms does not allow users, scientists and practitioners to access the code and the details of the available AR face filters. Scraping faces from these platforms to collect data is ethically unacceptable and should, therefore, be avoided in research. In this paper, we present OpenFilter, a flexible framework to apply AR filters available in social media platforms on existing large collections of human faces. Moreover, we share FairBeauty and B-LFW, two beautified versions of the publicly available FairFace and LFW datasets and we outline insights derived from the analysis of these beautified datasets.
OpenFilter Beautified Datasets
See our the detailed OpenFilter page for the generated beautified datasets.
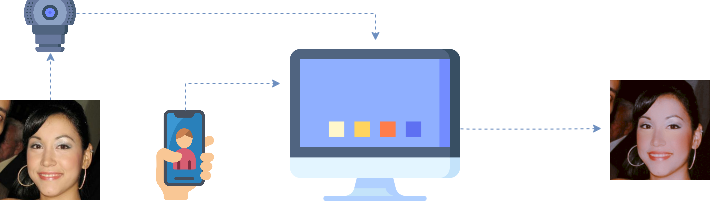